
Everything you ever wanted to know about Qualitative Comparative Analysis (QCA) but were too afraid to ask
While a fuzzy-set may sound like something you’d hear at a punk show, and a crisp-set might get your mouth watering, these are in fact, just two of the terms from the jargon filled world of Qualitative Comparative Analysis (QCA).
While there are few QCA practitioners in Australia (but with an active community in Europe and some other spots around the Globe), ARTD Director Brad Astbury has been experimenting with QCA since 2010 when he first came across the approach while investigating innovations in theory-based approaches to impact evaluation. In conversation with Brad, we dip into his knowledge and experience using this approach and set of techniques for case-based comparative research and evaluation.
First a bit of background
Developed by sociologist Charles Ragin in the late 1980s, QCA has since been applied to a range of fields from political science to construction, engineering and evaluation. QCA is both an approach (i.e. logic/strategy) and set of techniques for systematic cross-case analysis involving a small to intermediate sample of cases (typically between 10 and 50). It seeks to integrate the best features of qualitative and quantitative research to provide rich explanations of how, why and under what circumstances change occurs in the social world. (See the books and publications of Charles Ragin).
Why & when to use QCA?
QCA’s strength as a method lies in its ability to allow researchers and evaluators to “generalize findings across a relatively limited number of cases”, combining qualitative and quantitative methods to help “determine causal relationships between ‘causal conditions’ (similar to independent variables) and ‘outcome conditions’ (similar to dependent variables) [1].
In QCA, causality is viewed as complex and context sensitive. The goal is not to identify a single explanatory model that fits the data best, as is often the case with standard statistical techniques. Instead, the evaluator adopts a ‘case’ rather than a ‘variable’ view of analysis. This involves specifying for each case different combinations of conditions and outcomes and comparing across multiple cases to identify the most critical configurations of conditions that lead to particular outcomes.
What are the key steps?
Three variations of the method have been developed to deal with different research questions and data: crisp-set (csQCA), which converts qualitative data into binary categories; and fuzzy-set (fsQCA) and multi-value (mvQCA) both of which are designed for use with data requiring finer or more continuous categorisation.
Different sources on QCA differ in the number of steps they break the process down into, but the ones Brad tends to use are:
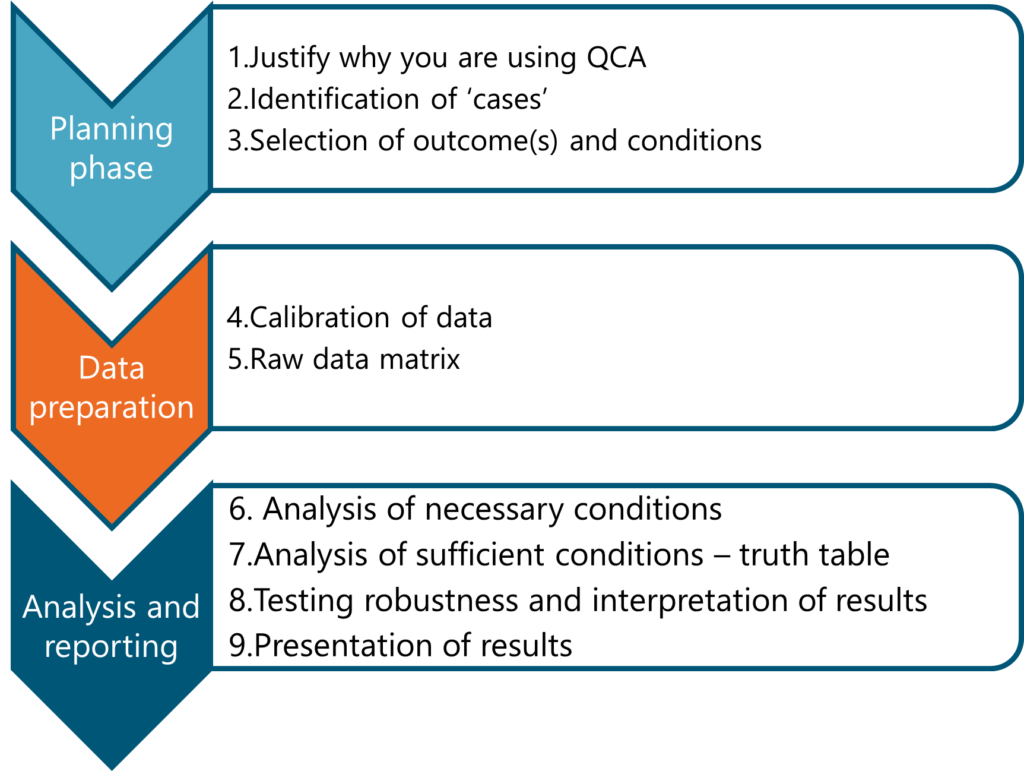
Comparative research and evaluation summary
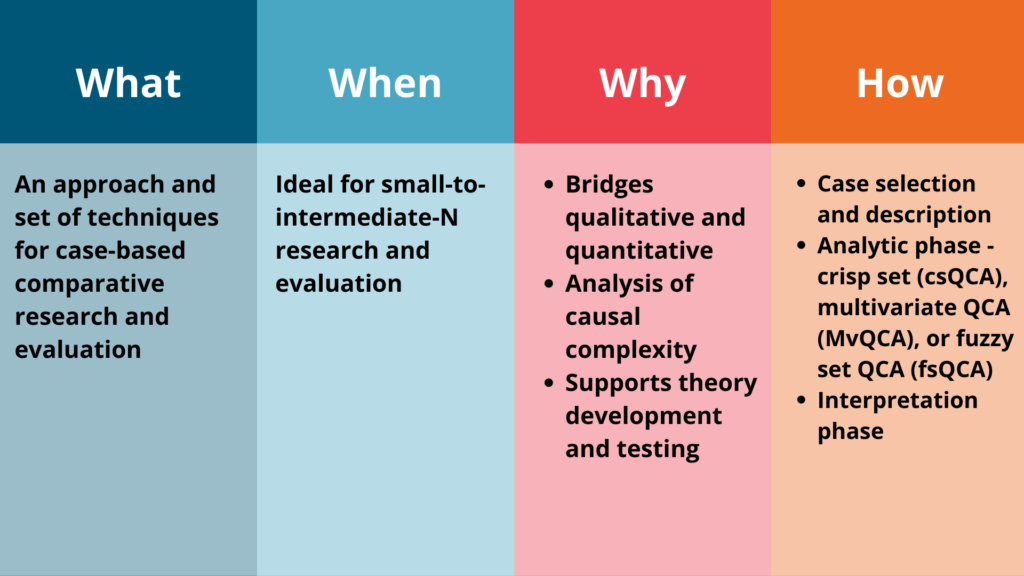
Watch this video with Brad Astbury for more on QCA, when it is most appropriate to use and the challenges and benefits of using it.
Additional information on key steps
Calibration of data
In this step scores are assigned for the outcomes and conditions based on triangulation of evidence for each of the cases (using a rubric can be very helpful here). Data is used to inform ratings of high and low achievement of outcomes, and conditions and the degree of membership in the chosen number of conditions that are hypothesised to influence the outcomes. Depending on whether the values are binary, fuzzy or multi-value, a scheme is then used for the calibration process where scores are applied, where 1=fully in and 0=fully out (there may be scores in between which denote ‘more in than out’ and ‘more out than in’ if dealing with fuzzy-set or multi-value).
Raw data matrix
Following calibration of conditions and outcomes, the next step is to prepare a data matrix of raw outcome and condition scores for each case. This is a significant preliminary stage prior to formal analysis and will require considerable engagement with empirical data from the cases to appropriately apply scores using the explicit criteria developed for evaluating degree of membership in each condition (Step 4).
A truth table is then constructed (this can be done using fsQCA software) with raw data based on membership scores for conditions and outcomes. A truth table is a synthetic display that enables rigorous exploration of different causal recipes that may be operating when a given condition is present or absent.
While the truth table is useful in terms of identifying which combinations of conditions are associated with outcomes, counterfactual comparison of case configurations helps to simplify the data into a minimal solution. The principle of Boolean minimisation underlying this process rests on the idea that configurations may differ in only one condition, yet produce the same outcome. In these instances, it is important to distinguish which conditions and their combinations are irrelevant or superfluous and remove these from the expression to create a more precise solution.
Standard analysis in fsQCA software applies an algorithm to generate solution outputs that differ in terms of assumptions made about unobserved configurations. These outputs represent the different causal pathways that are sufficient for ensuring the outcome.
References and resources
- Jordan, E., Gross, M. E., Javernick-Will, A. N., & Garvin, M. J. (2011). Use and misuse of qualitative comparative analysis. Construction Management and Economics, 29(11), 1159-1173.
- Ragin, C. C. (1987). The comparative method: Moving beyond qualitative and quantitative strategies. Berkeley, CA: University of California Press.
- Ragin, C. C. (2000). Fuzzy-set social science. Chicago: University of Chicago Press.
- Ragin, C. C. (2008). Redesigning social inquiry: Fuzzy sets and beyond. Chicago: University of Chicago Press.
- Rihoux, B., & Ragin, C. C. (Eds.) (2009). Configurational comparative methods: Qualitative Comparative Analysis (QCA) and related techniques. Thousand Oaks, CA: Sage.
- https://compasss.org/